Detection of Consciousness by Electroencephalogram
and Auditory Evoked Potentials
The article Detection of Consciousness by Electroencephalogram and
Auditory Evoked Potentials is a clinical investigation into the potential
for using electroencephalogram (EEG) and auditory evoked potentials
(AEPs) as parameters for demarcating between conscious and unconscious
states based on responsiveness or unresponsiveness to command. Both
of these recordings are accessible from the scalp and are thus non-invasive
and easily applicable in a surgical suite. EEG readings reflect spontaneous
cortical activity, whereas AEPs reflect activity from both the cortical
surface and from deeper regions of the brain, which suggests AEPs
are of value in the question of demarcation. Therefore, the investigators
in this article chose to explore what indicators or parameters in
EEG and AEP readings if any might serve as affirmation of unconscious
versus conscious states.
The issue the article principally tackles is that accurate interpretation
of EEG and AEP readings related to conscious states requires extensive
experience and exposure to cases of both consciousness and unconsciousness.
For example, AEPs require the measurement of peak latencies and amplitude
increases or decreases. Thus there have been some attempts at reducing
processed information from AEP and EEG into simplified numerical values.
For example, bispectral index monitors measure EEG readings and electromyographic
readings as well and thereby produce a numerical value that is suppose
to be indicative of the depth of anesthesia. The research in this
article however uses a set of EEG and AEP parameters based on statistical,
spectral, entropy, and chaos analysis in an attempt to identify a
set of parameters that allows demarcation of consciousness from unconsciousness
at the transition between them.
All the patients selected for the study were either normal healthy
patients (ASA physical status I) or patients with mild systemic disease
(ASA status II). They were all scheduled to undergo elective surgery.
20 patients received anesthesia with sevoflurane and remifentanil
(group 1) and another 20 received total intravenous anesthesia with
propofol and remifentanil (group 2). Non-invasive measurements of
vital signs and oxygen saturation, end-tidal carbon dioxide, etc.
were monitored with a Datex AS/3 monitor. The EEG and AEP electrodes
were applied and the results were digitally recorded on a personal
computer.
During anesthesia administration, each patient’s circulation
to the right forearm was occluded for 5 minutes in order to retain
ability to move the hand after succinylcholine was given. Once the
patient was unresponsive to the command to move the right hand (loss
of consciousness 1), the patient was then intubated and propofol or
sevoflurane administration ceased until the patient could follow the
command (return of consciousness 1). Next, sevoflurane or propofol
recommenced and once the patient was non-responsive to commands (loss
of consciousness 2), surgery was performed. After the surgery patients
were asked every 30 seconds to squeeze the investigator’s hand.
Return of consciousness 2 was defined as the first verified response
to command. Again, EEG and AEP were continually digitized and recorded.
Subsequently, the EEG recordings were processed and ran through a
converter, which allowed for a bispectral index machine to produce
the correlated numerical value based on the EEG. Ultimately, this
investigation delineated 19 EEG Parameters and 23 AEP parameters that
were assessed for indicators of consciousness versus unconsciousness.
The resulting analysis of these parameters was used in a logistical
regression to statistically calculate the probability of consciousness
under several mathematical models for selection of the best indicators.
The statistically derived best parameters for the combined EEG and
AEP data set contained a full model with sensitivity of 72.2% and
specificity of 85.3% with 15 AEP parameters and 4 parameters from
EEG with a probability of predictive success of 0.89. The best minimal
model had sensitivity of 75.4% and specificity of 84% with only 2
AEP parameters and two EEG parameters with a probability of predictive
success of 0.87. Analysis of the bispectral values calculated from
the replayed EEGs yielded only a 0.737 prediction probability.
In conclusion, this investigation ultimately shows that a combination
of parameters taken from EEGs and AEPs can out perform the bispectral
index value as a predictor between conscious or unconscious states.
In addition, with the predictive success of their minimal model, the
investigators show that the considerations for parameters must be
inclusive of at least two AEP parameters wavelet coefficient 11 from
detail level 3:db3_d3_11 and coefficient 5 from detail level 4:db3_d4_5,
and the two EEG parameters of median frequency of amplitude spectrum
between 8 to 30Hz and approximate entropy values. Their results for
these particular parameters are shown in adapted table below for summary.
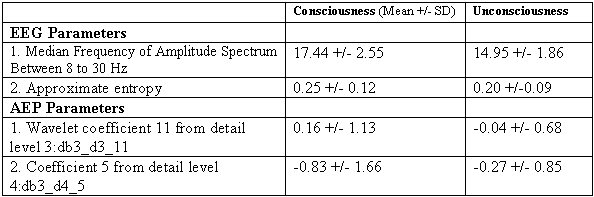
Click here for larger
image
Ray S. Magill - 11/05